Deep learning models have consistently been used to make groundbreaking advancements. Their application in predicting diseases from retinal images is one of them. A research team at Harvard Medical School have established deep learning techniques that have successfully analyzed retinal images for indicative signs of various diseases.
Processing retinal images into translatable diagnostic data introduces a promising approach to predict the risk of eye and systemic diseases. This approach could very well revolutionize the field of preventative healthcare and disease detection. By making use of artificial intelligence, it can provide a cost-effective pathway to monitor an individual’s health status.
These advances in retina-based diagnostics have the potential to give rise to predictive health care. It will have a profound effect on establishing preventive measures and ensuring timely interventions. Thus, reducing the impact of disease on the patients and healthcare systems.
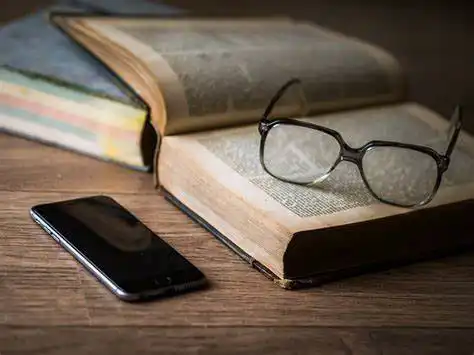
The deep learning model is trained to identify specific features in retinal images that are indicative of disease. For instance, changes in retinal blood vessels could provide early signs of diabetes, hypertension, and even Alzheimer’s disease. This consolidated approach in diagnostics opens up several opportunities to preserve health and save lives.
The retina serves as a window to the body's vascular health. The processes happening in retinal blood vessels resemble those in other parts of the body. As such, specific changes identified in retinal images could point to the likelihood of systemic diseases.
Training the AI models to predict disease risk from retinal images is a challenge. The team uses the method of Path Retinal Image Analysis (PRIA) to identify and quantify lesions in the retinal layers. The system predicts the risk score and likelihood of a person developing a disease.
The accuracy of these predictions depends upon the quantity and quality of retinal images. The more pictures available for analysis, the higher the accuracy of the data yielded by the algorithms. This robust application of deep learning techniques can help in the early detection of many diseases.
Predicting an individual's chance of developing systemic diseases like diabetes or cardiovascular disease through retinal images has been quite the scientific breakthrough. Recognizing these diseases early can prevent or delay their progression, providing significant health benefits for patients.
Issues such as diabetes, hypertension, cardiovascular disease, Alzheimer’s disease, and stroke, have significant symptoms in the retina. Hence, routine eye check-ups and retinal imaging could be a way to monitor general health and provide early detection of the diseases.
The research team is now working on furthering the diagnostic capabilities of retinal imaging. They aim to refine and improve their developed algorithms to make more accurate and timely predictions. More studies are in the run to make this procedure more reliable.
One of the advantages of utilizing deep learning models in healthcare is its ability to continuously learn and improve over time with the intake of more data. Hence, with more retinal images for analysis, the accuracy of these disease predictions is set to improve.
Accurate predictions can significantly impact how preventive measures for diseases are undertaken and how early interventions are carried out. Therefore, the utilization of retinal images can give rise to a new era of proactive healthcare.
While promising, this technological advancement in disease prediction is not without hurdles. There are myriads of facto+rs that affect a person's health - genetics, lifestyle, age, environment, etc. Including these factors into the machine learning algorithm to improve prediction accuracy is something the research team needs to work on.
The privacy of an individual's health information also poses another challenge. Balancing the benefits of predictive health care with the need to protect individual privacy will require careful consideration. Ethical issues and data usage regulations are also essential criteria in the application of this technology.
Furthermore, researchers need to address the potential for algorithmic bias in their machine learning models. This bias could result from a skewed distribution of data samples. Addressing this bias is crucial to ensure the fairness of the healthcare service provided to individuals.
In conclusion, such advancements in disease prediction signify an astronomical leap in the domain of healthcare. If adroitly cultivated, these deep learning models will establish a new standard of proactive healthcare. It will provide a cost-effective pathway to monitor an individual's health status, preventing catastrophic health scenarios at early stages.
This revolutionizing breakthrough needs to provide accurate, equitable, and ethical diagnostics. Merging this technology into the healthcare systems will undoubtedly lay the foundation for a healthier future. A future where individuals and healthcare providers make informed decisions about disease prevention and treatment.
In the ultimate analysis, the goal is to create a world where diseases are predicted and prevented before they cause significant harm. Thus, the application of deep learning models to retinal images contributes towards making this vision of proactive healthcare a reality.
While there are still challenges to overcome, the potential benefits of utilizing deep learning models for disease prediction are encouraging. If embraced by the healthcare industry, it could significantly change how we approach health and wellness.