Artificial Intelligence has become a significant tool in the field of climate change studies. It aids scientists in analyzing the enormous and complex data that characterize the effects of climate changes on human and environmental systems. Machine learning algorithms are helping researchers uncover hidden patterns in the data, significantly enhancing our understanding of these effects. This has opened up new directions in environmental and climate science studies.
The University of Illinois’ Grainger College of Engineering is pioneering these advancements in technology. The researchers have developed machine learning models, known as random forest models to interpret the outcomes of climate-related events. They have studied several factors including temperature, precipitation, and remotely-sensed data as part of these efforts. Combined with traditional statistical analysis, these methods have brought forth new perspectives on the impacts of climate change.
This research has led to significant results including the discovery of the relationship between crop yield and climate change. The team, led by Kaiyu Guan and Shaowen Wang, identified this relationship using the data analytics tools developed in their lab. They complemented this with on-the-ground observations to verify the findings. The complex interactions were well-captured by the machine learning algorithm, something not possible with traditional methods.
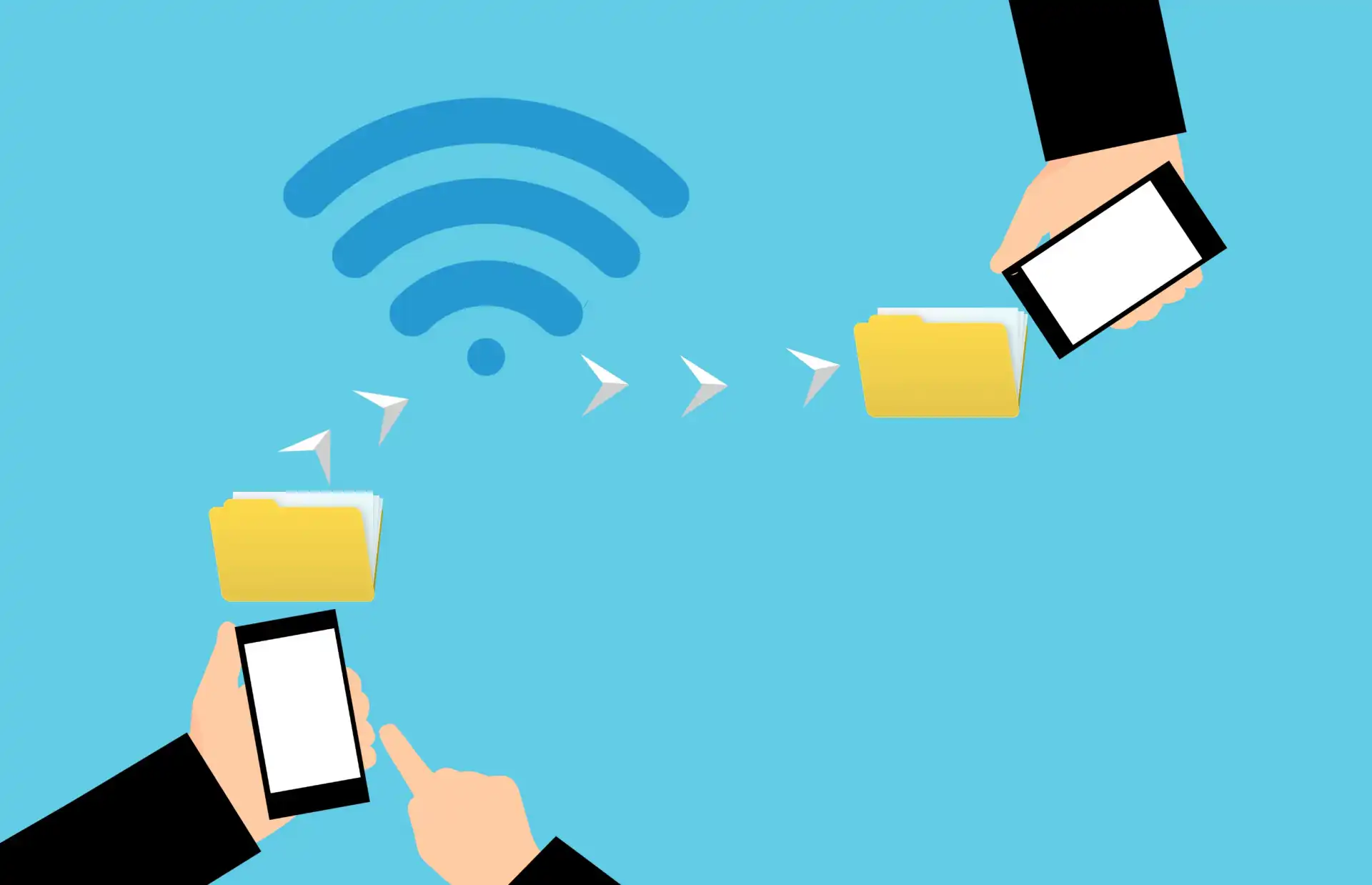
Similarly, another study from the University of Illinois’ School of Earth, Society, and Environment highlighted the role of machine learning in managing water resources. The application of machine learning helped them understand how climate-induced changes in precipitation patterns affect the groundwater resources. The insights derived from the pattern recognition can considerably contribute to water management strategies.
The introduction of advanced algorithms has revolutionized environmental science research. Machine learning allows scientists to sift through the huge amounts of data collected, eliminating the time-consuming procedures of traditional analysis. Furthermore, these tools have proven effective in identifying the statistical significance of climate variables on Earth’s natural systems.
As we understand more about climate change effects, this technology provides crucial assistance in policy-making. These findings can support the formation of sustainable solutions in sectors such as agriculture and water resource management. Policymakers can use these insights for planning out initiatives that help mitigate the impacts of climate change.
The research faucet into the immense potential of machine learning and Artificial Intelligence. The positive reception towards these technologies is integral in the formulation of new scientific inquiries. As more useful insights are discovered, we continue to figure out how we can utilize these advanced tools to mitigate climate change effects.
Notwithstanding the merits, the innovative data analytics method is not without its concerns. The interpretability of machine learning's results is a key challenge that researchers are seeking to overcome. This obstacle is significant because the correct interpretation of the outputs is a prerequisite for their utility in climate studies.
Another challenge is the ‘Black Box’ problem. In machine learning, the process in which the system learns to arrive at a particular conclusion isn’t always transparent. This ‘opaque’ nature of machine learning models often limits their application in climate science research.
Altogether, these challenges point to a growing need for a balanced approach. Researchers need to consider both the potential and limitations of these technologies when incorporating them into their studies. Integration of machine learning algorithms and traditional statistical models seems to provide a promising way forward.
The university researchers are making significant strides in addressing these concerns. They’re refining the random forest model, an algorithm that is a combination of decision trees, for more in-depth analysis. This is aimed at not only increasing the interpretability of the results but also augmenting transparency in the system.
In doing so, the researchers are paving the way for the optimal use of machine learning in environmental sciences. On account of their improved model, scientists can analyze the complex effects of climate change in a more comprehensive manner. It’s seen as a significant contribution to the modernization of data analytics methodologies in environmental research.
This progress in technology has undoubtedly had important implications for environmental science research. The application of machine learning in understanding and managing climate change effects could potentially initiate a new era of 'tech-driven' research in the field. The possibilities are endless and truly exciting.
The phenomenon of climate change is daunting and complex to comprehend. However, the use of advanced tools like machine learning simplifies the processes involved in understanding its impacts on Earth’s natural systems. From being a part of the problem, technology is becoming an essential part of the solution.
Scientists anticipate a promising future for machine learning in climate research. The dearth of profound understanding about certain unspecified effects of climate change can be tackled with advanced data analytics tools. Such technology brings an added dimension of aware decision-making in environmental research which was earlier beyond our reach.
Moving forward, we can expect a greater reliance on such technologies in environmental and climate science research. As we continue to grapple with the unfolding crisis of climate change, the integration of these innovative techniques will offer new ways to investigate the multifaceted challenges that it poses.
In sum, the advent of machine learning and AI in studying climate change is a groundbreaking development in environmental science. Confronted with the grand challenge of mitigating the effects of climate change, we now have a technique that could accelerate our efforts to comprehend and manage the impacts of this global phenomenon.
While a lot of work remains to be done, the future looks promising. Equipped with advanced technological tools, scientists are well-poised to uncover new findings and insights in the battle against climate change. As a community, our readiness and willingness to embrace this potential could prove critical in our quest for a sustainable future.