The University of Massachusetts Amherst researchers devised a groundbreaking machine learning model capable of predicting road accident spots based on specific roadway characteristics. It's an innovative approach that could enhance road safety and save lives.
The study, published in the journal Accident Analysis & Prevention, used Massachusetts State Police records to construct the model. The model will then sift through data on road curvatures, nearby amenities, speed limits, and the like to anticipate crash sites.
This new machine learning model's potential is extensive. It could go a long way in preventing collisions and mishaps by providing useful information to concerned departments such as city planners, transportation officials, and policy makers.
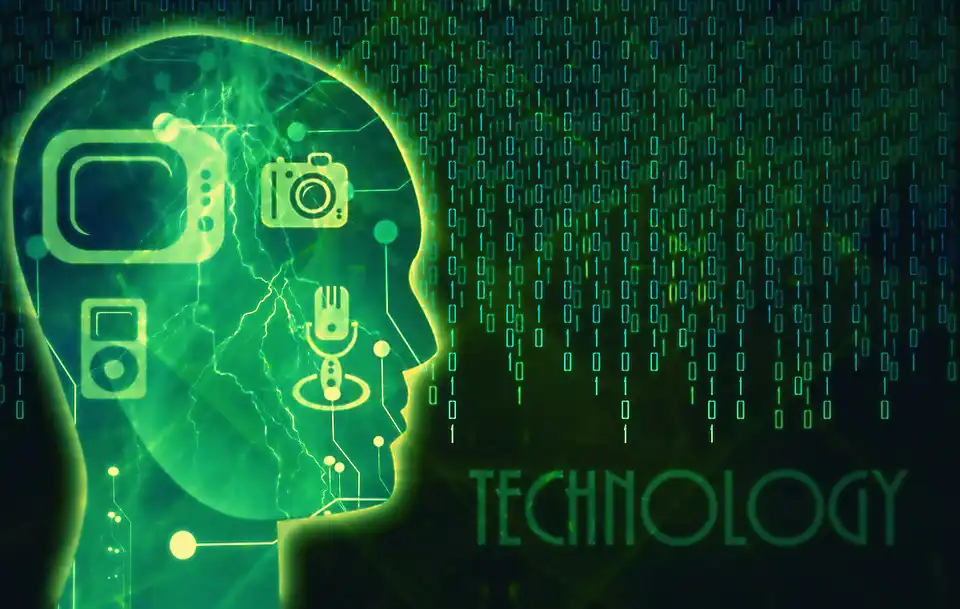
Database compilation was the initial step in this study. Researchers collected nearly three million crash records across Massachusetts over a decade. All the collisions recorded entailed property damage, injuries, or, sadly, fatalities.
Features of nearby roads were also collected. After all, characteristics such as curvature, speed limit, a school nearby, etc., all play a part in where accidents may happen. The team was careful to factor in a variety of possible elements.
After accumulating the necessary data, it was time for the machine learning model to take over. The model used is known as a 'random forest.' It creates an 'ensemble' of decision trees to form predictions.
And predict it did. With an impressive 90% specificity. That means, out of every ten predictions it makes about where crashes might occur, nine of them are likely to be correct.
One notable finding from the study was the impact of amenities close to roads. Roads near liquor stores, for example, had significantly higher accident rates. The study also found higher rates of accidents near distinct road features such as curves.
The machine learning model showed that accident rates were lower around speed limit signs. This serves as an important reminder of the role that speed limit signs play in ensuring the safety of the road.
Interestingly, the presence of a school both increased and decreased accident rates. Vehicle crashes were fewer around schools most of the day. However, during arrival and dismissal times, the number of accidents increased.
The way policymakers respond to these findings could have significant implications. For instance, they could push for more speed limit signs to be placed along the road to prevent accidents. In areas of high-risk, they could prioritize instituting traffic calming measures.
Cities could also adjust their transportation networks based on these findings. The data could further be used to adjust bus routes and schedules to reduce accident risks around schools during peak hours.
This is innovation at its peak, bringing together technology and data in a meaningful way. Not only does it have direct applications in urban planning, but it's also a major step forward in machine learning research.
However, it’s vital to note that the model isn't failsafe. It doesn't track real-time variables like weather conditions, or predict human behaviors — both of which significantly affect crash rates. But nevertheless, predicting road collision locales with a 90% success rate is an astounding achievement.
The use of machine learning in roadway safety analysis is still a relatively new field with considerable potential. There are many variables that could be factored into the models in the future which may improve their predictive abilities.
Another area for future exploration could be extending the model's use to other states or even countries. This would involve adjusting the model to consider different local factors, potentially increasing the model's effectiveness even further.
Still, the model's success rate is impressive. It's a testament to the potential of machine learning to play a significant role in enhancing road safety. When employed effectively, technology can truly save lives.
In conclusion, the University of Massachusetts Amherst researchers' study is a leap in the right direction. It’s a pioneering exploration into using machine learning to predict accident-prone areas. The model's effectiveness has the potential to revolutionize how we approach traffic safety.